The voices of Tax Policy Center's researchers and staff
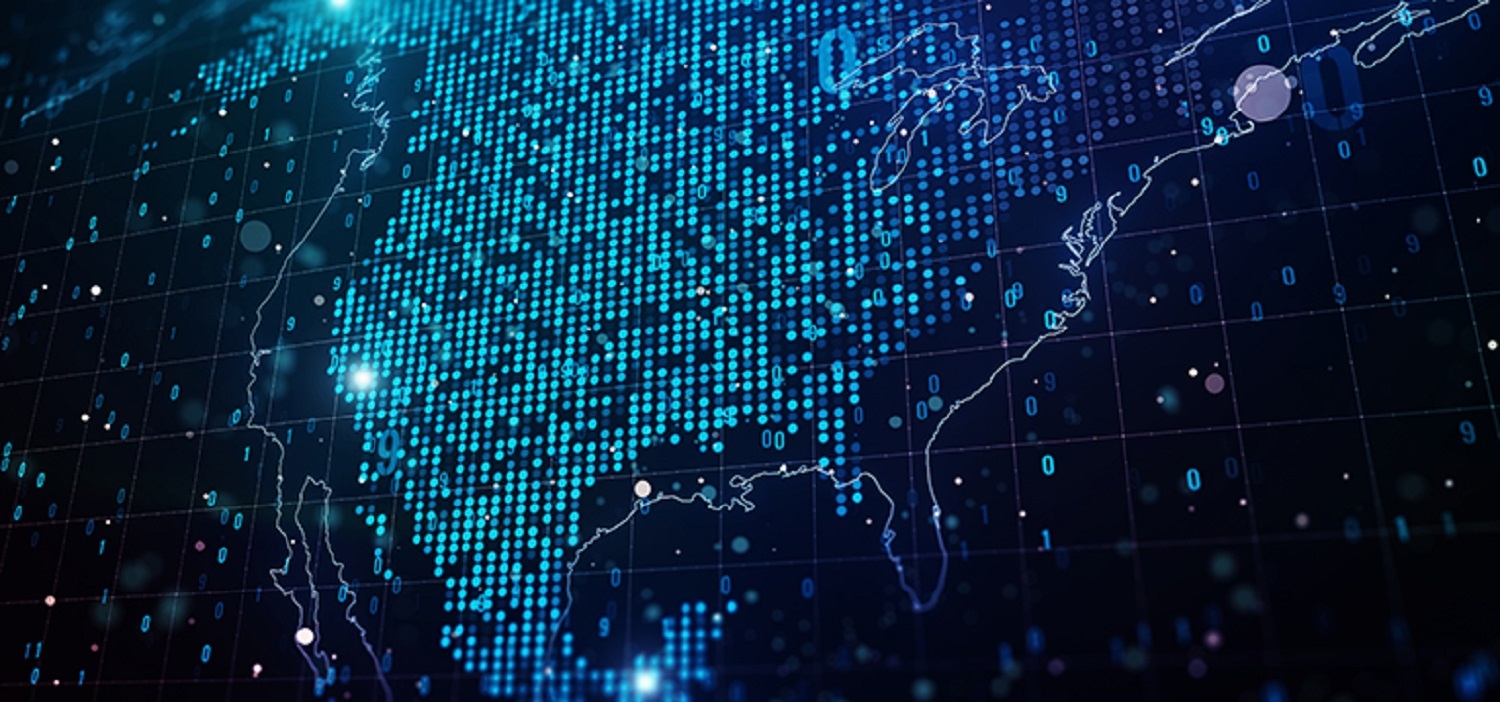
The synthetic control method (SCM) is a relatively new and valuable tool that makes it possible to measure state or local policy against a theoretical baseline, when a real basis for comparison doesn’t exist. Because the technique is constantly evolving, we wrote “An Update on the Synthetic Control Method as a Tool to Understand State Policy.” It incorporates some changes that improve the SCM, as well as some graphic intuition behind the method and a new way for visualizing how to select an ideal pool of potential controls.
The SCM was popularized by MIT economist Alberto Abadie and colleagues in 2010, when they used it to estimate how a tobacco tax increase in California affected cigarettes purchases. Some of its appeal comes from its ability to combine the clarity and transparency of a case study with the rigor of statistical analysis. The model has been widely used and many improvements have been added over the past decade.
In 2017, Rob and former TPC staff member Sarah Gault wrote “The Synthetic Control Method as a Tool to Understand State Policy.” Our new report updates that 2017 paper.
Analysts can use the SCM to evaluate policies that affect only a single “unit” or jurisdiction, such as a country, state, or city, where there isn’t an obvious control against which its experience can be compared. They create a synthetic control by combining and weighting a small number of jurisdictions that are similar to the one they are studying.
If the synthetic control is similar enough, researchers can understand the impact of a policy change by plotting the difference between the outcomes of the actual jurisdiction and the synthetic control. For example, in 2010 Abadie and colleagues estimated the effect of a 25 cent per pack increase in tobacco taxes in California by comparing cigarettes sales in that state with sales in their synthetic California.

Before the 1988 tax increase, per capita cigarette sales in synthetic California closely matched sales in actual California. But after the tax increase, actual sales fell faster than those in the synthetic California. That difference shows the estimated effect of the tax increase.
Our new report revises TPC’s 2017 guide to account for recent improvements in the SCM. For example, it now shows how to reduce interpolation bias, which can occur if the jurisdictions making up the synthetic control are not similar enough to the jurisdiction with the policy change. Perhaps surprisingly, this can occur even if the synthetic control is very similar to the jurisdiction with the policy change.
Although analysts often prefer large datasets, using a very large pool of potential comparison jurisdictions with the SCM can also bias estimates of a policy’s effectiveness. There are complicated ways to correct this problem, but we show a simple graphical method. We also provide links to computer code that incorporate some model improvements.
Given the SCM’s current popularity, analysts are increasingly likely to use it. Our updated guide is an easy-to-understand explanation of improvements in the SCM that can make the model more usable by researchers and policy analysts.
Posts and comments are solely the opinion of the author and not that of the Tax Policy Center, Urban Institute, or Brookings Institution.